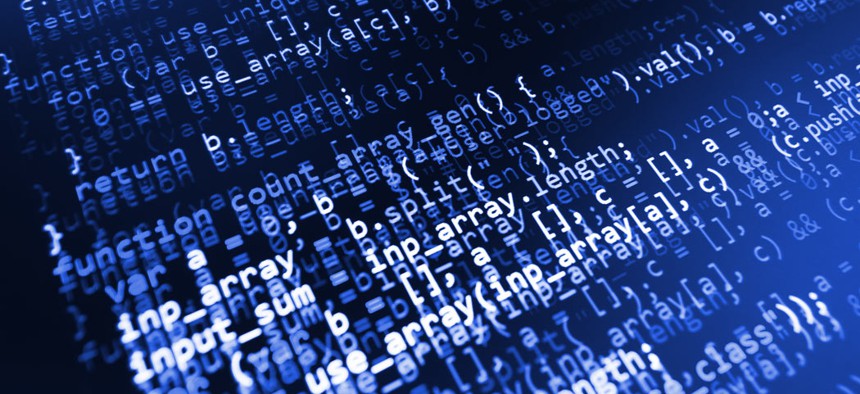
Pentagon research chief: AI is powerful but has critical limitations
DARPA director Arati Prabhakar cautioned against artificial intelligence as a panacea to problems that still need a human element.
Artificial intelligence has gained serious traction within the technology community as a solution for complex problems, though the head of the Pentagon’s top research arm is cautioning against it as a panacea.
“At DARPA today when we look at what’s happening with artificial intelligence we see something that is very, very powerful, very valuable for military applications, but we also see a technology that is still quite fundamentally limited,” Arati Prabhakar, director of the Defense Advanced Research Projects Agency, said at the Atlantic Council on May 2.
One example Prabhakar provided as a critical limitation is in image analysis. While artificial intelligence and machine learning systems are statistically better than humans at identifying images – and sifting through thousands of images in seconds – “the problem is that when they’re wrong, they are wrong in ways that no human would ever be wrong,” she said, providing a picture of a baby holding a toothbrush that a machine identified as a baseball bat. “I think this is a critically important caution about where and how we would use this generation of artificial intelligence.”
Many people within government have urged making more use of automation and intelligent systems to increase efficiency as data sets get exponentially bigger and as a way to operate at “cyber speed.” “We have organizations and machines that are capable of sharing information automatically, but … we need more machines to be able to automatically ingest it and act on it,” Philip Quade, special assistant to the director for cyber for the NSA’s Cyber Task Force, said last month.
Researchers have already developed cognitive systems to help humans sift through large data sets and identify objects of interest, such as mines beneath the ocean’s surface. Such developments will become ever more important as DOD increases use of unmanned systems. One example: upping the number of unmanned aerial ISR sorties by nearly 50 percent by 2019. “So if you look at what we collect in the Air Force, we used to collect [megabits] but now we’re collecting terabytes of data every day. It’s the equivalent of – just in full motion video – of two NFL seasons a day and analyzing it all,” Lt. Gen. Robert Otto, deputy chief of staff for Intelligence, Surveillance and Reconnaissance for the Air Force, said in February at an AFCEA NOVA luncheon. “My predecessor talked about we’re swimming in sensors and drowning in data but that’s only true if you can’t analyze everything.” For Otto, tagging metadata and leveraging automation and big data analytics will better enable human’s operations.
“There’s a criticism about the intelligence services are not connecting the dots. I think of how big data might be able to change that equation – connect dots that I’m not even thinking about – and then once we connect enough dots, it rises to a level that it hits a trigger that says, ‘Hey Bob, you should look at this,’ and then we can put our attention where it needs to go,” he said, noting that the situation is similar for the intelligence community.
Prabhakar acknowledged the high hopes for big data and analytics in optimizing human performance but was cautious in the capabilities of machines to provide all the answers. “I’m having trouble imagining a future where a machine will sort of tell us what the right thing is to do,” she said.
But that doesn’t mean improvements in AI can’t be very useful. “Now, of course at DARPA when we see those limitations we think, ‘Gee that’s going to be the next opportunity to drive the technology forward,’” she said. “So today, the other thing that we’re doing in addition to applying the first and second waves of AI is making the investments that we hope will create that third wave of artificial intelligence in which machines can explain themselves to us and tell us what their limitations are in which they can help us build causal models of what’s happening in the world. Not just correlations, but understanding causality in which they start learning how to take what they’ve learned in one domain and use it in different domains, something that they can’t really do at all today.”
For Prabhakar, deploying AI will have to be in the right place at the right time. “We have to be clear about where we’re going to use the technology and where it’s not ready for prime time, where it’s not really ready for us to trust it,” she said. “I think it’s just important to be clear-eyed about what the advances, in for example, machine learning can and can’t do,” she said, offering two simple examples: Artificial intelligence can be useful in the aerial domain countering a new radar signal and providing friendly aircraft with a new jamming profile immediately, while a self-driving car that needs to make determinations based upon sophisticated image understanding might be “imperfect in some dangerous ways.”