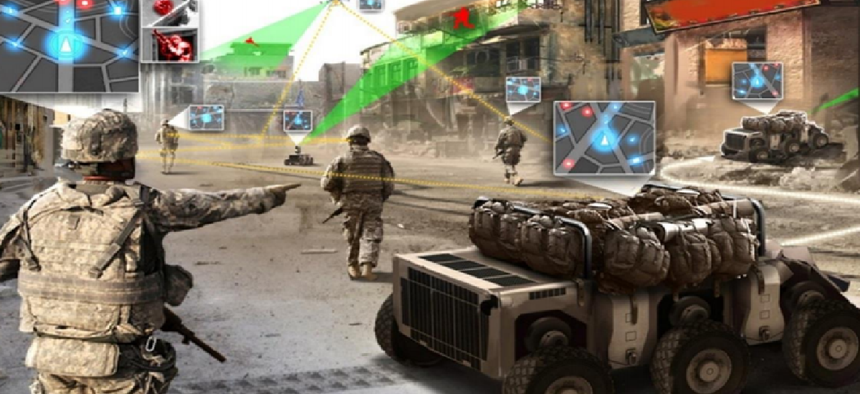
Back to basics: How this mindset shapes AI decision-making
A fighter pilot's decision-making principle -- observe, orient, decide and act -- can help military leaders apply leading-edge technologies to today's challenges.
When it comes to future military readiness, 2019 has been the year of artificial intelligence. The Department of Defense launched its AI strategy in February followed by the White House's executive order on “Maintaining American Leadership in Artificial Intelligence” -- both of which indicate accelerated delivery of AI-enabled capabilities and scaling the technology across DOD while cultivating a much-needed tech workforce.
Military leaders recognize AI’s potentially seismic impact on their mission and operations, and they expect practical applications to proliferate, from threat monitoring to asset tracking to predictive maintenance. Where, when and how those applications evolve from idea to reality is an unfolding story. So too is the global AI landscape, as Russia, China and other countries make substantial investments in such capabilities.
Given the urgency of the opportunities and threats posed by AI, it’s both fitting and somewhat ironic that a tested, decades-old military decision-making framework would offer a structure for commanders and executives to apply these leading-edge technologies. That structure is observe, orient, decide and act, or OODA.
AI and decision making
Every officer in the U.S. military has encountered the decision-making cycle, often referred to as the OODA (observe, orient, decide, and act) loop, popularized by U.S. fighter pilot Col. John Boyd. When paired with AI, it provides commanders and executives a practical, high-level framework to consider potential military and national security applications for the technology:
Observing (sensing). Weapons, vehicles and people are crucial data sources for the military. Every soldier, sailor or flyer is a potential sensor for internet-of-things application. So too are military assets such as ships, battlefield equipment and aircraft, when integrated into existing systems or designed into new ones. In this context, observing can fit into three categories. The first is data whose value does not justify real- or near-real-time acquisition; this data can be captured in bulk for later analysis. Second is data that warrants investment in retrofitting systems to achieve timely capture. Third is data valuable enough to warrant designing sensing capabilities into new armaments, devices, systems and other potential data sources.
Orienting (sense-making). Digital data becomes valuable when it can be understood. While hype continues to build around the potential for massive general AI deployment, narrow AI applications represent a sweet spot for the technology today. Industries such as trucking and railroading already use machine learning models extensively for predictive maintenance to anticipate and determine the root causes of failures. Our military has begun using AI on a limited basis as well and could benefit from much wider and faster deployment going forward.
Deciding and acting. Like orienting, AI holds potential for massive application in long-term decision-making and operationalization of chosen action plans. At the same time, significant near-term impact is possible through narrow and practical decide-and-act initiatives, such as the use of robotic process automation to streamline financial management and other labor intensive processes. These applications often emerge from an evolutionary process. A major trucking company, for example, began its AI journey by using the technology for sensing and sense-making. Over time, it began to integrate AI into new vehicles as an IoT application.
Targets for predictive action
Where should military leaders focus their AI efforts? Broadly speaking, all major combat weapon systems -- surface, sea, air and space -- can benefit from AI. As for where to start, systems that warrant priority status include those that meet two criteria: they are combat-essential with respect to high-priority plans and contingencies and they have the greatest potential readiness problems.
One such example is the Navy’s F/A-18 E/F Super Hornet fleet, which lost effectiveness due to insufficient mission-capable aircraft. Using machine learning, Navy leaders are gaining insights into how they can address underlying readiness problems and increase the availability of mission-capable strike fighters.
The Navy is also using AI to address the critical issue of aviation fuel quality. Machine learning is enabling examination of fuel composition down to molecular levels to identify chemical compositions that could trigger operational failures. These insights are dramatically reducing the time it takes to determine whether suspect fuels can be burned -- directly improving operational readiness.
Looking ahead, acting urgently
The military is beginning to make effective use of AI for observing and orienting. Logically, the next frontier is applying it to deciding and acting.
It’s one thing to build a predictive model that shows how improved parts management can increase aircraft availability for Super Hornet squadrons. It’s another to integrate such a schema into a combat unit’s decide-and-act flow. Accomplishing that at scale will require integration of AI capabilities into the military's operational, supply and enterprise resource planning systems.
Looking to the future, human-machine collaboration will become more possible and important to fighting and winning. The time will come when both battle networks and weapon systems employ AI to make some decisions and take actions on their own.
Defense leaders recognize the challenges AI presents and the resulting need for the services to make urgent changes at global scale. Realizing AI’s potential will drive them to decide where and how to apply the technology to operational combat systems and business enterprise operations. Winning tomorrow’s fight depends on faster and better decisions and actions today. Commanders and executives can lead mission-focused AI using a familiar framework -- Boyd’s OODA loop.