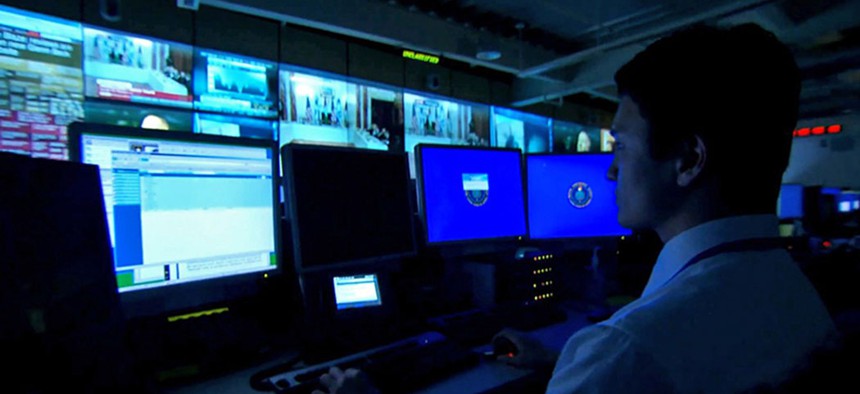
An official with the Defense Intelligence Agency works at one of the watch centers at headquarters. Defense Intelligence Agency via Wikimedia commons
What Happens When Spies Can Eavesdrop on Any Conversation?
The possibility of searchable conversations anywhere, thanks to better speech recognition software, recording device miniaturization, and future smart dust. By Patrick Tucker
Imagine having access to the all of the world’s recorded conversations, videos that people have posted to YouTube, in addition to chatter collected by random microphones in public places. Then picture the possibility of searching that dataset for clues related to terms that you are interested in the same way you search Google. You could look up, for example, who was having a conversation right now about plastic explosives, about a particular flight departing from Islamabad, about Islamic State leader Abu Bakr al-Baghdadi in reference to a particular area of northern Iraq.
On Nov. 17, the U.S. announced a new challenge called Automatic Speech recognition in Reverberant Environments, giving it the acronym ASpIRE. The challenge comes from the Office of the Director of National Intelligence, or ODNI, and the Intelligence Advanced Research Projects Agency, or IARPA. It speaks to a major opportunity for intelligence collection in the years ahead, teaching machines to scan the ever-expanding world of recorded speech. To do that, researchers will need to take a decades’ old technology, computerized speech recognition, and re-invent it from scratch.
Importantly, the ASpIRE challenge is only the most recent government research program aimed at modernizing speech recognition for intelligence gathering. The so-called Babel program from IARPA, as well as such DARPA programs as RATS (Robust Automatic Transcription of Speech), BOLT (Broad Operational Language Translation) and others have all had similar or related objectives.
To understand what the future of speech recognition looks like, and why it doesn’t yet work the way the intelligence community wants it to, it first becomes necessary to know what it is. In a 2013 paper titled “What’s Wrong With Speech Recognition” researcher Nelson Morgan defines it as “the science of recovering words from an acoustic signal meant to convey those words to a human listener.” It’s different from speaker recognition, or matching a voiceprint to a single individual, but the two are related.
Speech recognition is focused more precisely on getting a machine to understand speech well enough to instantly transcribe spoken words into text or usable data. Anyone that’s ever used a program like Dragon Naturally Speaking might think that this is a largely solved problem. But most automatic transcribing programs are actually only useful in very few situations, which limits their effectiveness in terms of intelligence collection.
It seems like an easy challenge for a military in the process of outfitting robotic boats with lasers, but speech recognition, especially in diverse environments, is incredibly difficult despite decades of steady research and funding.
A Brief History of Teaching Machines to Listen
The United States military, working with Bell Labs, launched research into computerized speech recognition in World War II when the military attempted to use spectrograms, or crude voice prints, to identify enemy voices on the radio. In the 1970s, IBM researcher Fred Jelinek and Carnegie Mellon University researcher Jim Baker, founder of Dragon Systems, spearheaded research to apply a statistical methodology called “hidden Markov modeling,” or HMM, to the problem. Their work resulted in a 1982 seminar at the Institute for Defense Analysis in Princeton, New Jersey, which established HMM as the standard method for computerized speech recognition. Various DARPA programs followed.
HMM works like this: Imagine you have a friend who works in an office. When his boss comes in late, your friend is more likely to come in late. This is a so-called Markov chain of events. You can’t observe whether or not your friend’s boss is in the office because it’s information that’s hidden from you. But when you call your friend and he tells you he’s not on time you can make an inference about the tardiness of your friend’s boss. Applied to speech recognition, the hidden state might be the thing actually being said but the clues are the sounds that commonly occur together.
Hidden Markov modeling has been the standard methodology for speech recognition for decades. Some noted scholars in the field like Berkley’s Nelson Morgan argue that reliance on it is now holding the field back. After all, while facial recognition has advanced tremendously enabling programs to detect faces and match them to databases in an ever-wider number of circumstances, speech recognition has not progressed nearly so well.
“In short,” Morgan wrote, “the speech recognition field has developed a collection of small-scale solutions to very constrained speech problems, and these solutions fail in the world at large. Their failure modes are acute but unpredictable and non-intuitive, thus leaving the technology defective in broad applications and difficult to manage even in well-behaved environments. In short, this technology is badly broken.”
One the most important characteristics of this dysfunctionality is what’s called a lack of robustness.
Mary Harper, program manager in charge of the ASpIRE challenge, explained the problem to Defense One this way: “Most speech recognition systems are trained to work for specific recording conditions. For example, a system trained on speech recorded in a conference room with an acoustic tile ceiling and heavy drapes using a high fidelity microphone won’t work very well on speech recorded in an unfurnished room with no sound-absorbing wall or floor coverings using a different type of microphone."
What form might those approaches take? Nelson in his paper suggests that today’s leaps in computational neuroscience, which have given rise to a number of interesting artificial intelligence applications like Siri, could be applicable to the speech recognition problem.
“There is an existing significant example of speech recognition that actually works well in many adverse conditions, namely, the recognition performed by the human ear and brain. Methods for analyzing functional brain activity have become more sophisticated in recent years, so there are new opportunities for the development of models that better track the desirable properties of human speech perception,” he writes.
Once speech data has been rendered as text it’s effectively been structured. That means it becomes far more workable as a dataset, allowing algorithms to crawl it in the same way the Google Search algorithm crawls the text of the world’s web pages. That small breakthrough doesn’t sound like much but it could actually revolutionize information gathering for the intelligence community. In theory, when speech in more different types of environments can be collected and transcribed any conversation happening within ear-shot of a networked microphone could become searchable in real-time.
For the intelligence community, achieving that sort of capability would require, in addition to better speech recognition software, the ability to collect speech data almost everywhere, particularly in contested areas where the U.S. has no boots on the ground.
But getting data collection devices into more places becomes easier with every iPhone purchase, thanks, in part to the Internet of Things. The next wave of interconnected consumer gadgets like Google’s Moto X superphone and the Apple Watch coming in 2015 represent a broad trend in devices that rely on voice commands and speak to users, as Rachel Feltman points out in a piece for Defense One sister site Quartz. Are the voice commands that you give your future smart watch legally open to intelligence gathering?
The defeat of the U.S.A. Freedom Act means that the National Security Agency can continue to collect meta-data on cell phone users, which can be used to pinpoint location. Depending on where you talking to your device, whether in public or in private, a judge may rule you don’t have a reasonable expectation of privacy. But if you’re worried about your device becoming a listening ear for the government, so, too, could the very air around you.
Shhh… The Smart Dust Will Hear You
The intelligence community in the decades ahead will rely on an ever smaller and capable array of microphones to pick up intel and some border on the unbelievable. Scientists have actually created a microphone that is just one molecule of dibenzoterrylene (which changes color depending on pitch.) Devices that pickup noise or vibrations can be as small as a grain of rice.
Continued advancement in the field of device miniaturization could one day allow for the dispersal of extremely small but capable listening machines, one of the uses a future technology sometimes called “Smart Dust.”
What is the strategic military advantage presented by ubiquitous, tiny listening machines? In a 2007 paper (PDF) titled Enabling Battlespace Persistent Surveillance: the Form, Function, and Future of Smart Dust, U.S. Air Force Major Scott A. Dickson speculates that future micro-electromechnical systems or MEMS will “sense a wide array of information with the processing and communication capabilities to act as independent or networked sensors. Fused together into a network of nanosized particles distributed over the battlefield capable of measuring, collecting, and sending information, Smart Dust will transform persistent surveillance for the warfighter [sic].”
The nascent opportunity to turn the physical world into a landscape for surveillance is a theme that’s showing up with growing frequency in scholarly defense literature, such as this September 2014 paper out of National Defense University’s Center for Technology and National Security Policy, which heralds the future opportunities that the Internet of Things provides for the “monitoring of individuals and populations using sensors.”
Before researchers arrive at a searchable soundscape, better speech recognition will help efforts in speaker recognition, attaching a specific voice in a recording to a specific person. IARPA says that speaker recognition isn’t the goal of the current challenge. But that sort of capability has clear and near-term applications for national security.
In more and more conflict areas, big investments in facial recognition are revealing themselves to be of very limited use. Consider Ukraine, where fighters carefully kept their faces hidden from international observers while effectively annexing another country’s territory. Or think of northern Iraq, where jihadists committing barbaric acts do so, often, under mask.
Every time a new video from the Islamic State surfaces, intelligence workers are faced with the challenge of matching the voice of the person in the video to that of someone else, someone who once walked the streets. Doing so means having a wide sample of voices to compare to the one in the video.
Today, companies and law enforcement agencies routinely collect so-called voiceprints on customers and suspects. In 2012, the FBI announced a technology called VoiceGrid to store voice data. Today, the Federal Police in Mexico have a database of more than a million voice records taken during criminal proceedings and arrests. But the number of voice prints potentially available to law enforcement or the intelligence community surpasses 65 million by some recent estimates. As large as that number sounds, it will likely grow exponentially as speech recognition, speaker recognition and device miniaturization advance.
It’s a trend with clear privacy implications. But the reliance of groups like the Islamic State on anonymity speaks to an intelligence challenge that will persist in the coming decades. War is changing, whether it is waged by emergent groups like the Islamic State or nations like Russia, more and more, the potential revelation of identity is becoming a liability in conflict zones. Knowing the name of the person on the other-side of the battlefield is rising as a strategic necessity. That’s what makes continued bugging of the world inevitable.