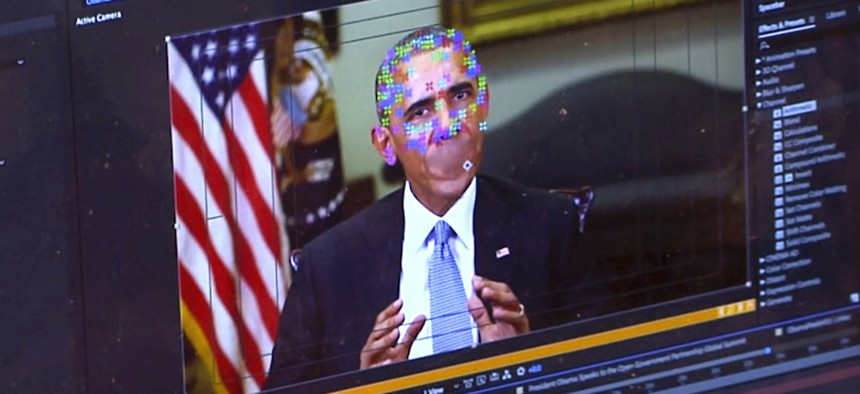
This image made from video of a fake video featuring former President Barack Obama shows elements of facial mapping used in new technology that lets anyone make videos of real people appearing to say things they've never said. AP Photo
Deepfakes Are Getting Better, Easier to Make, and Cheaper
GitHub is becoming a destination site for make-your-own-deepfake software.
Deepfakes —computer-generated images and footage of real people — have emerged as a major worry among the national security set. A new paper from researchers at FireEye finds that tools published to open source repositories such as GitHub are reducing the amount of technical expertise required to produce ever-more-convincing deepfakes. Moreover, they are increasingly easy to purchase from disreputable marketing and PR firms.
In the paper published online today and presented (virtually) at the cybersecurity conference Black Hat, researchers Philip Tully and Lee Foster write that it takes thousands of dollars and weeks to produce new software tools for synthetic media generation. “However, the application of transfer learning can drastically reduce the amount of time and effort involved,” they write. In other words, a set of commonly-available tools has emerged that lends itself to the creation of faked images and voice recordings.
They mention a generative adversarial [neural] network or GAN model dubbed StyleGAN2, the underling code of which is available on GitHub. Tully and Lee showed that with a dataset of compiled Tom Hanks pics, structured to be of the same size and general direction, they could easily use StyleGAN2 tools to create new, convincing images of the actor, which they revealed in their Wednesday demonstration.
They next showed how easy it was to use software called Transfer Learning from Speaker Verification to Multispeaker Text-To-Speech Synthesis, or SV2TTS, to clone the voice of the actor to go with the fabricated images. Programmers have, similarly, contributed to the SV2TTS GitHub page. “All we need to do is collect some audio samples [of the intended target] which are freely available to record via the Internet, load up a few of the resulting M4A files into the pre-trained SV2TTS model, and use it as a feature extractor to synthesize new speech waveforms,” they write.
Researchers, including many with funding from the U.S. military, have been working on ways to automatically detect deepfakes using biometric signals (the fact that certain physical indicators like pulse don’t reproduce well in faked video) The good news from Tully and Foster’s research is that out-of-the-box deepfakes are relatively easy to spot, also using machine learning programs available on GitHub. But the more a would-be actor with money and time is able to customize the software or the underlying dataset, the harder it becomes to detect. “Detection accuracy dipped to around 78% for fine-tuned generations as the distribution of scores output by the classifier shifts closer to chance, as shown in red. So if threat actors were to fine-tune on a custom dataset they themselves collated, this could present a problematic asymmetry between the data used to create the synthetic generations and the data blue teams would have access to—or even knowledge of—with which to build a commensurate detection model,” they write.
In their presentation, they noted that some marketing firms had already begun to offer deepfakes as a service, with prices varying based on time spent and sophistication of the product. Very few of those firms included any safeguards to make sure that the deep faked footage was produced with the consent of the individual whose likeness was stolen.
As strategist Peter Singer has pointed out, the enormous volume of new, grainy, filmed-at-home video footage, over platforms such as Zoom, means deepfakes will be more difficult to stop simply because people are becoming more used to consuming lots of grainy, choppy, video-footage. “The quality bar does not need to be exceedingly high when it comes to synthetic generations; it only needs to be “good enough” for even just a subset of vocal users to not question it in a world characterized by rapid, high-volume information consumption.”
There’s a reason deepfakes are emerging as a major national security concern, impersonating groups like journalists is becoming an increasingly common tactic among key adversary groups. Pro-Iranian actors have impersonated journalists in order to solicit comments to produce pro-regime propaganda (in February, Facebook announced that they had removed several accounts based in Iran for that reason.) Russia, too, has taken to hacking legitimate news sites in order to push fake news stories aimed at undermining NATO in places like Lithuania, Poland, and Ukraine. Ultimately, easier-to-access deepfaking tools suggests that behavior will accelerate.
NEXT STORY: US Missile Defenses Are About to Level Up