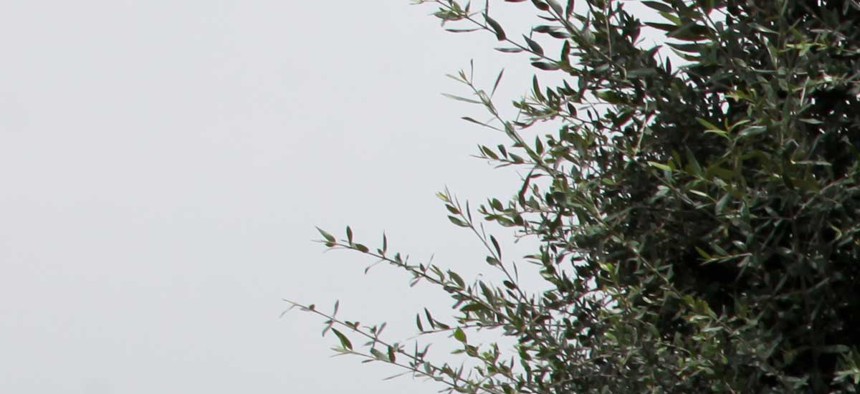
Soldiers assigned to the 501st Infantry Regiment speak with Afghan villagers of upcoming mortar fire in areas surrounding Combat Outpost Narizah, Khost province, on July 7, 2012. Defense Imagery Management Operations Center by Sgt. Kimberly Trumbull
Violence Is More Likely To Occur in Pro-US Villages in Afghanistan
That is one of many insights found in a new data project run by researchers at Yale University.
The United States’ efforts to “win hearts and minds” as it fought the Taliban in Afghanistan seem to have created a cruel and fatal paradox.
When political scientist Jason Lyall of Yale University in the United States surveyed the mood of villages strewn across the country’s southern provinces he found that those with the most pro-US feeling were the most likely to draw punishment attacks from the Taliban. Worse, the US was no more likely to find improvised explosive devices (IEDs) in those supportive villages.¹
The dynamics behind this are not totally clear. But the implication is that US efforts to win villagers’ hearts and minds were successful enough to render their villages Taliban targets, but not enough to convince them to provide useful intelligence about IEDs. If true, the military is thwarting its own aim, stated in the US Army Field Manual, of “creating safe spaces for the population by reducing insurgent attacks.”
It’s a suggestion so controversial that Lyall and his team are still working to convince themselves—and their paper’s peer-reviewers—that civilian attitudes could influence attack predictions so strongly.
But even at this early stage it’s a powerful example of the insights that the emerging field of violence forecasting could yield. That’s because Lyall’s results come from an algorithm that takes in information from village surveys and spits out predictions for where violence will occur.
Statistical and computer models that predict behavior might sound like science fiction, but several groups are doing similar research. In doing so they are identifying possible causes of conflict, raising hopes of prevention, and potentially providing guidance on safety and stability for development work.
Seeing further
The few existing efforts to predict violence typically use prior incidents to forecast future ones. To improve on this, in early 2011 Lyall’s team surveyed 2,754 men in 204 Afghan villages about their level of support for the Taliban and the International Security Assistance Force (ISAF). They combined this information with data on insurgent violence and the locations of military bases and aid projects.
The researchers built these factors into a statistical model. It showed that villages’ levels of support for ISAF predicted IED attacks occurring in the 15 km (9.3 mi) around each village for up to the next 10 months. A village showing “modest” ISAF support would suffer 13 extra attacks on average over the following five months than one strongly opposed to ISAF.
Lyall and his colleagues tested this relatively simple statistical model on another 14,606 villages they hadn’t surveyed. They combined data on previous incidents in these villages with attitude estimates extrapolated from the surveyed villages, and improved IED attack predictions by up to 30%.
Lyall stresses, however, that field tests must be run and peacekeepers and police need to cooperate more closely with researchers before they can trust these estimates enough to use them.
The survey itself cost around $150,000, which Lyall admits is high—but relatively cheap by the standards of surveys in places such as Afghanistan. Cheaper methods can be used once a model is built.
“Targeted surveys with purpose-built questions are likely to have a higher predictive payoff than large-scale surveys at a fraction of the cost,” Lyall says. He is also confident this approach could build prediction models for other types of violence.
Both the military and NGOs have shown an interest in the technique, says Lyall. “Being able to predict future violence would allow development agencies to select areas for greater aid effectiveness,” he explains. The Africa-centred Ushahidi collaboration’s crowd-sourced CrisisNet could provide ideal “micro-level data” for prediction, he says.
Deceived wisdom
Other research from Yale strengthens the case for using survey-based statistical predictions of violence.
In a paper undergoing peer-review, Yale’s Robert Blair and his colleagues predict violence such as murders and rapes in Liberia.² The team was already surveying 242 Liberian communities about violence and dozens of geographic social, and economic factors for a randomized controlled trial (RCT). After donors asked Blair’s team if the data could forecast violence, he found that one model correctly predicted 88% of violent incidents using just five variables.
One variable that appeared to be linked to violence was the prevalence of power-sharing agreements where minority ethnic groups were given a say in local governance. This is interesting because political scientists have traditionally recommended power sharing as a good way to help avoid conflict.
Blair’s models appear to cast doubt on this, much like Lyall’s team’s study does on the “hearts and minds” approach. Blair says that such models could help evaluate other received wisdom from politics about peace-keeping, as political science often lacks the means to test its explanations.
Collecting the data were “laborious, expensive and slow,” Blair admits, and the work must be replicated in each country before predictions can be made. However, he is optimistic that if and when others confirm their findings in Liberia, exploiting the results need not be expensive.
“Once you have an accumulation of studies, you can narrow down to fewer and fewer variables,” he explains. “Then you can design, for instance, a cellphone-based survey with local leaders every couple of weeks. That’s cheap.”
Blair is now working towards such projects with a consortium of NGOs and governmental organizations called the Early Warning-Early Response (EWER) working group in Liberia. EWER’s activities include the interactive Liberia Early-Warning and Response Network (LERN) map, developed by Ushahidi to detect and avert possible conflict.
“They have a lot of folks in the field but they don’t have a very systematic way to generate insights from observations,” says Blair. “We’re working to see how we can systematise that.”
The Liberia Early Warning and Response Network maps reports of violence and disturbances in the country. This map shows examples connected to ethnic tensions, but the network records reports related to several other categories including rape, looting and drugs.
Blair is now hoping to create violence-forecasting models using data from surveys in Indonesia and Iraq. He’s also involved with an effort to forecast violence on a very different scale, with the Political Instability Task Force (PITF) funded by the US Central Intelligence Agency.
They have a large data set, gleaned from a program that trawls the internet gathering information from the language people use online, Blair explains.
Although the Liberia data set is small by comparison, Blair believes it’s still competitive with this “big data” approach.
The PITF’s programs “vacuum the internet and code news stories into data points, but the algorithm is messy,” he says. “You don’t know to what extent you’re modeling noise.”
“We stick to the opposite approach. If an instance of violence occurs we’re going to get as positive as we can that it really did occur. Plus, in a place like Liberia there isn’t that much media online for algorithms to scrape. That’s probably true of places like Afghanistan, Sub-Saharan Africa and the Middle East—the places we care most about may be the places it’s hardest to get data on.”
Peace prizes
Another set of violence prediction efforts in the US has resulted from recommendations from the Genocide Prevention Task Force, co-convened by the United States Holocaust Memorial Museum in Washington, DC.
Based on this advice, the US government decided in 2012 to set up a cross-agency collaboration called the Atrocities Prevention Board (APB). As a member, the US Agency for International Development (USAID) asked itself whether the latest technologies were being used to prevent or respond to mass atrocities.
By October 2012, USAID had announced a competition intended to answer this and other questions. The Tech Challenge for Atrocity Prevention involved five sub-challenges, including a predictive modeling challenge.
The model challenge was a series of contests. “We first ran a ‘data hunt mini-challenge’ for good predictors of violence, and so people sent us ideas such as oil and food prices,” says Maurice Kent, USAID’s lead expert for prize competitions.
The agency then brought together these suggestions with PITF’s data, and the Global Database of Events, Language and Tone, a similar open-source collection of over 400 million global news events.
In March 2013, the agency set a competition to develop algorithms that would use this data to predict violence. It received 618 models from 100 contributors and in November 2013 awarded a $12,000 first prize for the model that made the best predictions, as well as four additional prizes. In order for the government to use the competing models later, if required, USAID acquired non-exclusive licenses to them under the terms of entry.
USAID ran another stage of its Tech Challenge for Atrocity Prevention in November 2014, awarding seed fund grants of up to $50,000 to previous winners who proposed partnering with an NGO to pilot or further develop their product.
Projects in four of five sub-challenges then received follow-on grants, but the model challenge had no funded projects.
“We didn’t get any applications,” explains Mark Goldenbaum, a human rights advisor for USAID. “That shouldn’t come as a surprise as it was one of the more difficult ones to field test.”
But USAID is making the 618 models available to the public, and one of the competition’s judges, Jay Ulfelder from the Holocaust Memorial Museum’s Early Warning Project, hopes to use them.
Plausible predictions
While the Early Warning Project also originated from Genocide Prevention Task Force recommendations, it is not US government owned or funded. Instead, it is supported by the Holocaust Memorial Museum and Dartmouth College, who employed Ulfelder to get it operational.
Ulfelder says the project’s goal is to inform a global audience. “If we say ‘this country you weren’t thinking about actually looks relatively high risk,’ USAID can maybe say it’s a higher priority for conflict prevention. An NGO that funds projects to prevent violence may say ‘We weren’t planning on doing anything in this country next year, but now it’s time we move onto it.’”
The Early Warning Project is intended to produce real-time forecasts in two ways. The first is a risk assessment exploiting three models, two using statistical methods similar to the village-scale efforts.
“We train models on historical data, apply them to current data, and get a predicted probability of onset of state-led mass killing in a given year for all countries in the world,” Ulfelder explains. Initial assessments were published on the project’s blog in summer 2014.
While Ulfelder says he would “love” information as detailed as Lyall and Blair’s teams, surveys would be difficult to initiate in countries most at risk of state-led mass killings. “Some wouldn’t let you run a survey, because they’re authoritarian regimes,” Ulfelder points out.
“In some places practically it would be very difficult, as it was in Afghanistan, so the cost would be inordinate. Conceptually I’m thrilled to see what they’re doing, but it’s not going to happen any time soon for projects like ours.”
Ulfelder highlights that, whatever the scale, collecting large enough data sets is a big difficulty—something USAID’s other Tech Challenges seek to address.
“The problem is worst with tracking violence,” he says. “It’s basically impossible to observe political violence that people often want hidden, like deliberately killing civilians, for all countries in the world, all the time. Even if they’re not trying to hide it, often places at highest risk are the places where we’re least likely to see it.”
So for its real-time forecasts, the Early Warning Project uses cheap, coarse, publicly-available data from sources like the PITF, the World Bank and the International Monetary Fund.
The second prediction activity is an “opinion pool” of forecasts from experts on regional politics and genocide that became available when the Early Warning Project’s official website launched in February 2015.
“Our goal is to recruit hundreds of people,” Ulfelder says. “We’ve got about 125 right now but really want to grow those numbers from people all around the world, especially from where the risks are higher, to have their views informing the output and making it more useful.”
Ulfelder is also working on a proposal to continue crowdsourcing development of the models from the USAID competition, getting software developers among the general public to improve them further.
With the Early Warning Project close to full deployment, Ulfelder admits that it now faces another crucial test, shared by any attempt at prediction. It must convince potential users that its assessments are credible.
“Some people believe statistical analysis and modeling are trustworthy. Another sort of people routinely distrust those things,” he says.
“Any organization we talk to will have both. The challenge is making the information available to people inclined to trust it and enhance that trust—and persuade others it’s worth their attention.”
¹Kentaro Hirose and others, “Can Civilian Attitudes Predict Civil War Violence?” under review.
²Robert Blair and others, “Predicting Local Violence,” under review.
This post originally appeared at SciDev.net.