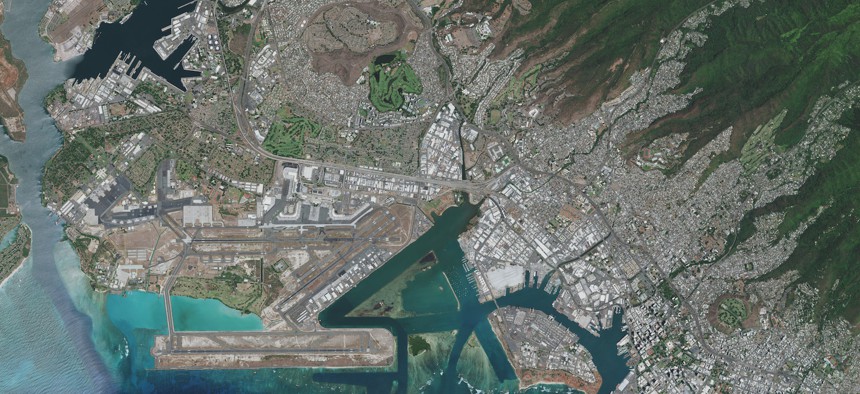
NOVEMBER 4, 2020: Maxar overview satellite imagery of Honolulu. Please use: Satellite image. This image is merely to illustrate "satellite imagery." It is not a faked image. (c) 2020 Maxar Technologies.
A Better Way to Spot Deep-Faked Satellite Images
Training AIs to look at 26 subtle features may help thwart attempts to peddle fraudulent imagery.
Computer-generated satellite “photos” can be very difficult for humans and other machine learning algorithms to detect, a growing concern of national security officials who fear that doctored images might find their way into troops’ hands or be used to sway public opinion. But help may be on the way. Researchers this week published a new method for detecting faked satellite images, even those that would normally fool advanced computer detection techniques as well as trained human eyes.
The team from the University of Washington started by creating the best fakes they could. Using a tool called CycleGAN, they created a generative adversarial network that pitted two artificial intelligence algorithms against one another. The first AI worked to spot fake images, and the second identified the factors that the first AI used to find the fakes and used those lessons to produce even more flawless frauds. Ultimately, the team created a set of 8,064 satellite images, including real images of Tacoma and Seattle, Washington, and Beijing — and faked ones that combined imagery of the three cities.
“With our own eyes, it was nearly impossible to tell whether the simulated satellite image was authentic or fake,” they wrote.
They then fed the images to a new machine-learning tool that takes into consideration 26 image features related to things like brightness, color channels, and more.
Their tool correctly identified 94 percent of the fake images. But it also mischaracterized several real ones as fake, achieving an overall reliability of 73 percent.
This “indicates that we can distinguish the fake satellite images by taking a closer look at their color, edge clarity, and texture characteristics,” they wrote.
The challenge of spotting faked satellite images is neither small nor inconsequential. In 2019, Todd Myers, automation lead for the CIO-Technology Directorate at the National Geospatial-Intelligence Agency, or NGA, described the threat at a Defense One event: “from a tactical perspective or mission planning, you train your forces to go a certain route, toward a bridge, but it’s not there. Then there’s a big surprise waiting for you.”
While NGA and government agencies are able to corroborate open-source imagery with classified information, the general public does not. That poses the risk of an adversary releasing doctored images of conflict lines, troop deployments, etc. to create misleading public narratives about where troops might be massing.
“The Chinese are well ahead of us,” he said at the time.
The FBI last month warned that they anticipate much greater use of deep fakes in the months ahead.