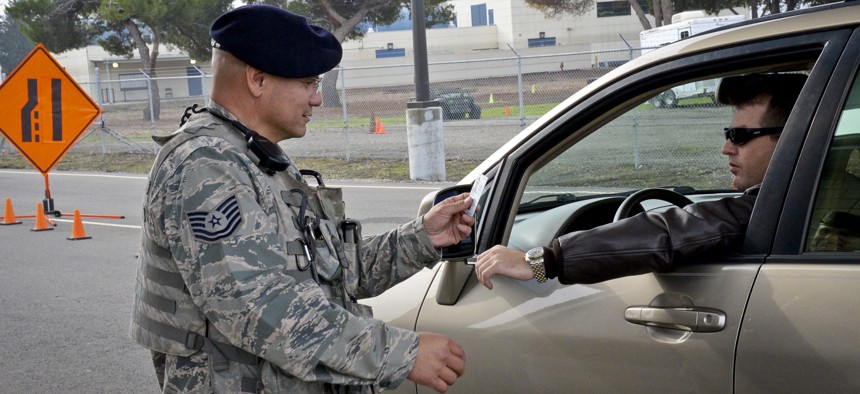
A California Air National Guard NCO checks an idenitification card at the main gate to Moffett Federal Airfield, Calif., on Dec. 8, 2014. U.S. California Air National Guard photo by Tech. Sgt. Ray Aquino
To Prevent Insider Threats, DOD Must First Define ‘Normal’
The Pentagon thinks it can build an automated system to predict and prevent future Fort Hood-style attacks. But it’s a problem as big as big data itself.
On November 5, 2009, not long after 1 p.m., Army psychiatrist Maj. Nidal Hasan approached a long line of soldiers awaiting medical screening at a soldier readiness center at Fort Hood, Texas. After bowing his head in prayer, Hasan opened fire with a FN Herstal Five-seven semiautomatic pistol. Seven minutes later, 13 soldiers were dead and 33 more wounded, including Hasan.
Imagine your job is to anticipate when that sort of thing might occur by using digital data produced by and about soldiers like Hasan. The task before you is enormous. There were around 65,000 people personnel stationed at Fort Hood at the time of Hasan’s rampage. If you were to scan every piece of electronic communication between those soldiers, and their contacts, for hints of future violence, your graph would include 14,950,000 different nodes—people, devices, and other communication points—and up to 4,680,000,000 messages, according to a paper published after the incident by the Defense Advanced Research Projects Agency, or DARPA. Nevertheless, predicting such insider attacks on the basis of digital clues is something the Defense Department is committed to doing.
Following the 2011 leak of classified documents by Army Pvt. First Class Bradley (now Chelsea) Manning, President Obama signed Executive Order 13587, establishing the National Insider Threat Task Force . The Defense Department and the Task Force have since taken various steps to predict and prevent insider attacks. But the ultimate goal — a continuous and automated understanding of every potential threat posed by everyone with any connection to the defense establishment — remains elusive.
DOD is working to meet one of the Task Force’s key recommendations: establish a center to coordinate its insider threat efforts, codify best practices, and monitor the most important cases. This Defense Insider Threat Management and Analysis Center, or DITMAC, slated to reach initial capability this fall, is meant to be able to analyze and fuse insider threat data that it gets from different components. Full operational capability will come in 2018 or 2019.
Insider threat detection, as specified by DOD requirements, will also become standard for any defense contractor with top-secret clearance, according to forthcoming changes in the National Industrial Security Program Operational Manual.
Key to DoD’s these efforts is what’s called continuous evaluation, a method to continuously monitor personnel with important clearances or access for changes that Department believes could make them insider threats, like credit problems, family issues, arrests, etc. But DoD would like to be able to subject all personnel to that sort of examination.
A recent quarterly
report
of the Insider Threat program suggested that 225,000 personnel would be subject to continuous evaluation by one of the CE pilot programs by the end of 2015, and 1 million by 2017. But, as
recent GAO reports
make clear, progress has been uneven.
The DoD CE program will automatically and continuously check records using data from the government, commercial sources to insure to look for warning signs. “As it matures, the CE capability will continuously ingest and filter data as it becomes available and provide ... relevant information to appropriate security entities for action,” Mark Nehmer, who is leading that effort as the DITMAC team’s deputy chief for implementation, told Defense One in an email.
That means developing software to detect threat signals, bad credit, unusual travel, arms purchases, etc. the second that that information becomes available.
The usefulness of continuous evaluation will depend on DOD’s ability to tell normal behavior from abnormal on a department-wide scale and do so automatically. A few years ago, DARPA funded a program called Anomaly Detection at Multiple Scales, or ADAMS. The
goal
was to “create, adapt and apply technology to the problem of anomaly characterization and detection in massive data sets…The focus is on malevolent insiders that started out as ‘good guys.’ The specific goal of ADAMS is to detect anomalous behaviors before or shortly after they turn.” That set the stage for many current research efforts.
“We’re working through a number of different efforts right now, behavioral analytics, to actually try and figure out [for] all of these different known insider threats that we’ve seen, what the characteristics have been of those individuals, what are the behaviors that they’ve exhibited,” said Nehmer.
“Where were they in their work life? Where were they in their social life? We’re doing as deep a dive as we can get on individuals that have actually exhibited insider threat behavior,” Nehmer said at a recent Defense One event .
That seems straightforward enough — but it presents a technical challenge as big as big data. Consider Hasan, on whom the Army had an abundance of data. They knew that he had become withdrawn after receiving abuse from other soldiers and that he wanted out of the military. In the summer of 2009, they knew that Hasan had begun to buy non-issue guns .
The military looks for those behaviors among many others, as indicators of potential insider attacks. This 2011 guide to characterizing so-called “indicators of potential insider attackers lists a few of them.
“Socially withdraws on some occasions,” “strange habits,” and “lacks positive identity with unit or country” are Category I indicators that could apply to every American teenager. The Category III indicators include “takes unusual travel,” “Exhibits sudden interest in partner nation headquarters or individual living quarters,” and “Stores or collects ammunition or other items that could be used to injure or kill multiple personnel.” But these clues can present themselves too late.
The thing about “normal behavior” is that its definition depends entirely on context. Normal for one person in one job is abnormal for another. “There is no normal across a department,” says Nehmer, “There is no normal across a command … We’ve been doing some machine learning in the department and they’ve been looking at what normal behavior looks like by a single position. You go to a particular component, you go to a sub unit in that component, and then you look at a position. We know what that should look like. That’s where we’ve done the homework.”
“For instance, say you have someone who is an administrative assistant. And this assistant is spending a lot of time looking at foreign military websites. And they’re downloading things off the Internet and sending emails to a foreign email address. So someone looks into that and finds that the person is actually putting together travel for one of the flags [flag officers], in that particular country.”
Nehmer says that that sort of behavior should be automatically flagged by a user activity monitoring, or UAM, system, prompting a closer inspection by a human analyst within the insider’s component. It shouldn’t necessarily trigger high institutional concern. Developing software to automatically flag and possibly mitigate concerns as they come up will involve training programs on massive amounts of anonymized data related to emails, texts, and messages.
As continuous evaluation rolls out, a higher level of surveillance is going to become the norm across the Defense Department. Patricia Larsen, co-director of the National Insider Threat Task Force, said that while continuous evaluation might feel intrusive for DOD employees, it doesn’t actually represent a fundamental change from types of screening and vetting procedures that the Department undertakes now.
Analysts looking for insider threats today can use a tool called the Automated Continuous Evaluation System, or ACES , to run queries about an individual across different databases. It’s Google for instant background checks, not truly automatic.
“ACES has been running for 20 years,” Larsen said. “So we have been looking at you as a person. But it’s been a slow, methodical and sometimes painstaking way of doing it. What we’re saying is that there’s a lot of information about you that’s already out there. We want to put it together in one place so we can short-circuit the information gathering point and get to the analysis of you faster and be able to look at you more proactively.”
Carrie Wibben, director security policy and oversight division for the Office of the Under Secretary of Defense (Intelligence) likened the new level of scrutiny to a routine lie-detector test. “I don’t like that,” she said, “but I feel better knowing that everyone alongside me had to go through the same polygraphic examinations that I had.”
Of course, continuous evaluation will be a great deal more intrusive than a one-time polygraph session. It will, among other things, collect every DOD worker’s public posts on social media along with all other communication that the Department can legally collect. Such data could be used, as far as possible, to monitor not just credit and life changes but each individual’s current emotional state. “A machine could monitor that and get a contextual feel for a person’s mood. I think that’s in the future. But we have to sift through the volumes that we’re talking about and you have to get a baseline for people,” said Nehmer.
Deriving a sense of mood or sentiment from textual clues, perhaps in real time, is a fast-growing area of machine learning research.
Consider this paper by Chris Poulin in which he and his fellow researchers tried to use subtle language clues harvested from social media to predict which veterans might attempt suicide. They found indicators that allowed them to predict with better than than 65% accuracy.
Then there’s another worry: the data that’s left off the table. Asked how her office was looking into deep web or dark web content for insider threat indicators, Wibben answered, “We’re not looking to that at all. We have to stay within the bounds of the [Standard Form 86] consent form, so things that individuals put out there publicly, that’s what we’re looking at right now. If you protect it behind a password, we’re not looking at it… at least not on the background investigation, continuous evaluation side.”
All the data that DOD can obtain, legally but at no small inconvenience to those who soldiers who create it, may still not be enough to prevent future insider attacks from taking place.