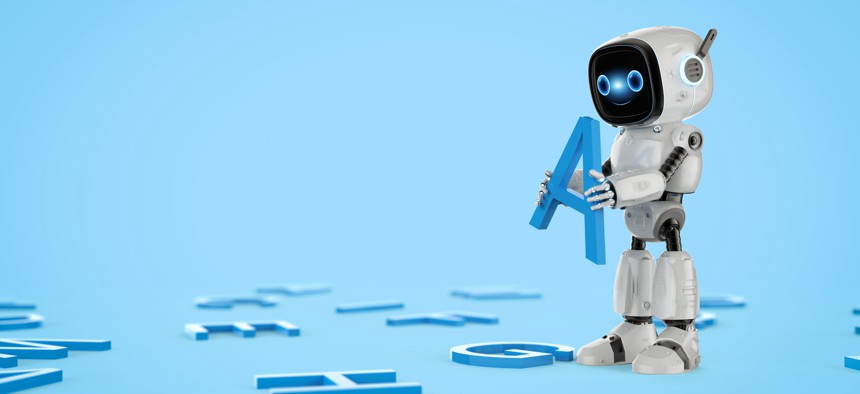
PhonlamaiPhoto
Don’t Neglect ‘Small-Data’ AI
Big-data artificial intelligence may prove unsuitable for defense applications.
Much hand-wringing has attended the notion that China has a “natural advantage” in the race to develop artificial intelligence because, as an autocratic state that casts a wide digital net, it is better placed to gather the vast swaths of data needed to train machine-learning models.
But big-data AIs are not the only AIs—and indeed, they may prove too data- and energy-intensive to undergird safe, reliable, and trustworthy AI-enabled defense technologies. Several new “small data” approaches promise better, quicker results—if the Pentagon ensures that they are not starved for funding in the race.
This was becoming apparent as far back as 2017. “The appropriate operational data can be difficult to obtain or lacking,” wrote Elsa Kania at the Center for a New American Security. "Even obtaining a comprehensive dataset to account for one’s own military is challenging.”
More recently, a 2021 report from Georgetown University’s Center for Security and Emerging Technology, or CSET, highlighted the diversity of AI techniques, including “small data” approaches, that the United States and China compete over closely as data becomes more valuable. CSET analysts Husanjot Chahal and Helen Toner wrote in Scientific American in favor of “transfer learning” —an AI approach that works with comparatively less data than mainstream techniques by starting with a large data set, then retraining the program “slightly using a smaller data set related to your specific problem.” This reduces the need for endlessly growing AI systems’ training data.
Another approach, known as “neuro-symbolic AI,” arises from a recognition that deep learning’s accuracy and reliability vary intolerably, even with massive datasets, as Don Monroe writes in Communications of the ACM. By seeking to combine deep learning with abilities inspired by human reasoning, neuro-symbolic AI promises more reliable systems as well as energy- and data-thrifty development.
AI experts Gary Marcus and Ernest Davis suggest that Meta’s new “Cicero” —the first AI to achieve human-level performance in the game Diplomacy—may possess elements of a neuro-symbolic AI. It is heartening to find AI researchers, like DeepMind’s David Pfau, acknowledging Cicero’s significance. It is not yet clear how applicable Cicero’s underlying design will be outside of Diplomacy. However, its surprising existence offers evidence that AIs incorporating multiple designs are possible in high-level domains.
Planning for small-data AI
Currently, most AI funding is geared toward machine-learning approaches. This may lead to a scenario in which there is a vast amount of public and private funding for AI research, but without a mechanism to capture the benefits of small-data techniques. This scattershot approach to the fusion of AI with military technology echoes a larger problem in scientific research: finding good ideas in a mountain of knowledge.
A better situation should be shepherded by the Defense Department’s fledgling Chief Digital and Artificial Intelligence Office, or CDAO, which integrates several defense AI-oriented teams in a bid to keep AI projects moving amid the Pentagon bureaucracy.
Such a plan might look like this:
Near-term: Big-data designs dominate, but targeted acquisitions are made in smaller-data approaches, like transfer learning, for applications requiring highly specific datasets. The CDAO signals to private companies that smaller data approaches will be valued.
Next: These smaller-data approaches begin to be integrated alongside existing deep learning applications, slowly moving the Defense Department away from a big-data mindset. New sets of targeted acquisitions are made in more refined, small-data techniques.
Finally: Small-data approaches like neuro-symbolic AI, which aim to replicate the efficiency of human data consumption, begin to be developed and integrated in place of existing AI applications, depending on the levels of reliability they afford. Deep learning and transfer learning, and other approaches, could exist alongside neuro-symbolic AI, using hybrid forms as much as reliability and efficiency allow.
The CDAO may find a useful institutional venue in the National Science Foundation, which has been authorized by last year’s CHIPS and Science Act to establish a technology, innovation, and partnerships directorate.
An incremental funding scheme, implemented for flexibility, is also worth consideration.
What if this plan does not work as intended? There are still benefits for the United States.
First, it puts substantive action behind the knowledge that AI is more than machine learning. Although the efficiency of China’s “military-civil fusion” policy is often exaggerated, China’s centralized system does have strategic benefits regarding data hoarding and AI development. But the idea that China has “a unique advantage based on its access to more data…and lack of privacy protections” is only true if big data AI is the only game in town. It is not.
Second, it allows the Defense Department to mitigate some of the problems and limitations with deep learning systems that are all too real, even if fully neuro-symbolic AI eludes researchers.
Finally, it puts resources behind American industry in contrast to the relative lack of faith China may have, for now, in its domestic industries. These recommendations balance the thought styles of futurists and traditionalists: recognize what works with deep learning, articulate what does not work, and make sure human talent is harnessed in carving out new pathways in AI and national security.
Vincent J. Carchidi is an analyst with RAIN Defense+AI. His opinions are his own.
NEXT STORY: When Lippy Generals Challenge Civilian Control