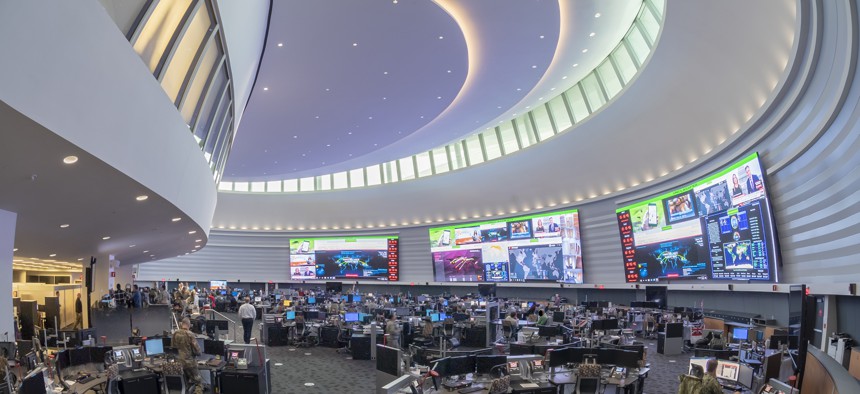
U.S. CyberCommand's Integrated Cyber Center and Joint Operations Center, or ICC/JOC NSA / US Cybercommand
Spies Like AI: The Future of Artificial Intelligence for the US Intelligence Community
Putting AI to its broadest use in national defense will mean hardening it against attack.
America’s intelligence collectors are already using AI in ways big and small, to scan the news for dangerous developments, send alerts to ships about rapidly changing conditions, and speed up the NSA’s regulatory compliance efforts. But before the IC can use AI to its full potential, it must be hardened against attack. The humans who use it — analysts, policy-makers and leaders — must better understand how advanced AI systems reach their conclusions.
Dean Souleles is working to put AI into practice at different points across the U.S. intelligence community, in line with the ODNI’s year-old strategy. The chief technology advisor to the principal deputy to the Director of National Intelligence wasn’t allowed to discuss everything that he’s doing, but he could talk about a few examples.
At the Intelligence Community’s Open Source Enterprise, AI is performing a role that used to belong to human readers and translators at CIA’s Open Source Center: combing through news articles from around the world to monitor trends, geopolitical developments, and potential crises in real-time.
“Imagine that your job is to read every newspaper in the world, in every language; watch every television news show in every language around the world. You don't know what's important, but you need to keep up with all the trends and events,” Souleles said. That's the job of the Open Source Enterprise, and they are using technology tools and tradecraft to keep pace. They leverage partnerships with AI machine-learning industry leaders, and they deploy these cutting-edge tools.”
AI is also helping the National Geospatial-Intelligence Agency, or NGA, notify sailors and mariners around the world about new threats, like pirates, or new navigation information that might change naval charts. It’s a mix of open source and classified information. “That demands that we leverage all available sources to accurately, and completely, and correctly give timely notice to mariners. We use techniques like natural language processing and other AI tools to reduce the timelines reporting, and increase the volume of data. And that allows us to leverage and increase the accuracy and completeness of our reporting,” Souleles said.
The NSA has begun to use AI to better understand and see patterns in the vast amount of signals intelligence data it collects, screening for anomalies in web traffic patterns or other data that could portend an attack. Gen. Paul Nakasone, the head of NSA and U.S. Cyber Command, has said that he wants AI to find vulnerabilities in systems that the NSA may need to access for foreign intelligence.
NSA analysts and operators are also using AI to make sure they are following the many rules and guidelines that govern how the NSA collects intelligence on foreign targets.
“We do a lot of queries,” NSA-speak for accessing signals intelligence data on an individual, Souleles said. Queries require audits to make sure that NSA is complying with the law.
But NSA technicians realized that audited queries can be used to train AI to get a jump on the considerable paperwork this entails, by learning to “predict whether a query is reportable with pretty high accuracy,” Souleles said. That could help the auditors and compliance officers do perform their oversight roles faster. He said the goal isn’t to replace human oversight, just speed up and improve it. “The goal for them is to get ahead of query review, to be able to make predictions about compliance, and the end result is greater privacy production for everyone.”
In the future, Souleles expects AI to ease analysts’ burdens, proving instantaneous machine translation and speech recognition that allows analysts to pour through different types of collected data, corroborate intelligence, and reach firmer conclusions, said Jason Matheny, a former director at the Intelligence Advanced Research Projects Activity and founding director of the new Center for Security and Emerging Technology at Georgetown University.
One roadblock is the labor of collecting and labeling training data, said Souleles. While that same challenge exists in the commercial AI space, the secretive intelligence community cannot generally turn to, say, crowdsourcing platforms like Amazon’s Mechanical Turk.
“The reason that image recognition works so well is that Stanford University and Princeton published Imagenet. Which is 14 million images of the regular things of the world taken from the internet, classified by people into about 200,000 categories of things, everyday things of the world; toasters, and TVs, and basketballs. That's training data,” says Souleles. “We need to do the same thing with our classified collections and we can't, obviously, rely on the world's Mechanical Turks to go classify our data inside our data source. So, we've got a big job in getting our data.”
But the bigger problem is making AI models more secure, says Matheny. He says that today’s flashy examples of AI, such as beating humans at complex games like Go and rapidly identifying faces, weren’t designed to ward off adversaries spending billions to try and defeat them. “Current methods are brittle,” says Methany. He described them as vulnerable to simple attacks like model inversion, “where you reveal data a system was trained on, or trojans, data to mislead a system,”
In the commercial world, this isn’t a big problem, or at least it isn’t seen as one yet, because there’s no adversary trying to spoof the system. But concern is rising, in 2017, researchers at MIT showed how easy it was to fool neural networks with 3D-printed objects by just slightly changing the texture. It’s an issue that some in the intelligence community are beginning to talk about as well with the rise of new tools such as general adversarial networks.
The National Institute of Standards and Technology has proposed an AI security program. Matheny said national labs should also play a leading role. “To date, this is piecemeal work that an individual has done as part of a research project,” he said.
Even a bigger problem is that humans generally don’t understand the processes by which very complex algorithms like deep learning systems and neural nets reach the determinations that they do. That may be a small concern for the commercial world, where the most important thing is the ultimate output, not how it was reached, but national security leaders who must defend their decisions to lawmakers, say opaque functioning isn’t good enough to make war or peace decisions.
Most neural nets “with a high rate of accuracy are not easily interpretable,” says Matheny. There have been individual research programs at places like DARPA to make neural nets more explainable. But it remains a key challenge.
New forms of advanced AI are slowly replacing some neural nets. Jana Eggers, CEO of Nara Logics, an AI company partnered with Raytheon, says she switched from traditional neural nets to genetic algorithms in some of her national security work. Unlike neural nets, where the system sets its own statistical weights, genetic algorithms evolve sequentially, just like organisms, and are thus more traceable. “Look at a tool like Fiddler,” a web debugging proxy that helps users debug and analyze web traffic patterns, she said. “They’re doing sensitivity analysis with what I would consider neural nets to figure out the why, what is the machine seeing that didn’t necessarily.”
But Eggers notes that making neural nets transparent also takes a lot of computing power, For all the different laws that intelligence analysts have to follow, the laws of physics present their own challenges as well.