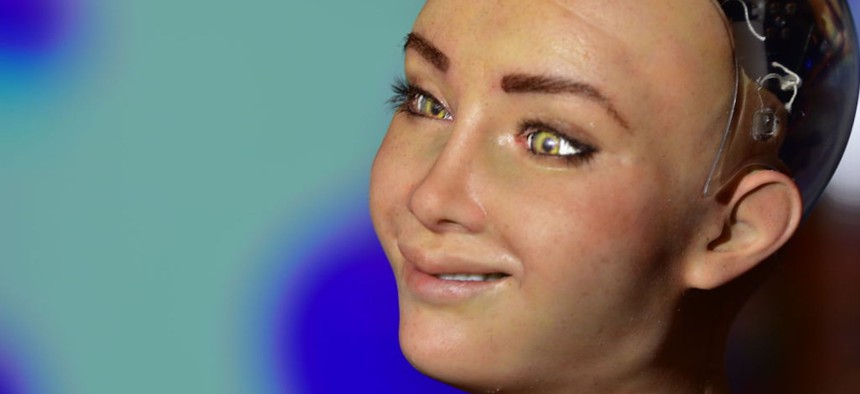
Sophia the Robot, who received citizenship from Saudi Arabia and made history as the first robot with an identity card, is introduced in Antalya, Turkiye on July 8, 2023. Fatih Hepokur / Anadolu Agency via Getty Images
AI is supposed to become smarter over time. ChatGPT can become dumber.
‘The real takeaway is that Large Language Models are unstable,’ one expert said.
AI models don’t always improve in accuracy over time, a recent Stanford study shows—a big potential turnoff for the Pentagon as it experiments with large language models like ChatGPT and tries to predict how adversaries might use such tools.
The study, which came out last week, looked at how two different versions of Open AI’s Chat GPT—specifically GPT-3.5 and GPT-4—performed from March to June. GPT-4 is the most recent version of the popular AI that came out in March;. Open AI described it as a huge improvement over the previous version.
“We spent 6 months making GPT-4 safer and more aligned. GPT-4 is 82% less likely to respond to requests for disallowed content and 40% more likely to produce factual responses than GPT-3.5 on our internal evaluations,” the company said.
But the Stanford paper showed GPT-4 performed less well than GPT-3.5 on difficult math problems—and that it actually got worse at math between March and June. “GPT-4’s accuracy dropped from 97.6% in March to 2.4% in June, and there was a large improvement of GPT-3.5’s accuracy, from 7.4% to 86.8%,” they write.
This is bad news for the military, for which continual improvement of large language models would be critical. Various senior Defense Department officials have expressed concerns and even terror at the thought of using ChatGPT for military purposes, because of the lack of data security and the sometimes bizarrely inaccurate results. But other military officials indicate an urgent need to employ generative AI for things like advanced cybersecurity. Improved accuracy across versions over time would likely eventually satisfy critics and lead to possible adoption—if not of ChatGPT itself, then similar models.
One of the benefits of generative AI is that it can be useful for writing code, even if the user has very limited programming knowledge. That’s a core concern for the U.S. military, which wants to put coders closer to combat.
Gen. Charles Flynn, who was the Army’s deputy chief of staff in 2020, told reporters at the time: “We have to have code-writers forward to be responsive to commanders to say, ‘Hey, that algorithm needs to change because it’s not moving the data fast enough.’”
But while making coding easier would be a big advantage for frontline operators, the Stanford researchers discovered that both GPT-4 and GPT-3.5 produced fewer code samples that could simply be plugged in immediately (or “directly executable.”) Specifically “50% of generations of GPT-4 were directly executable in March, but only 10% in June,” for GPT-4, with similar results for GPT-3.5.
GPT-4 also uses far fewer words to explain how it reached conclusions. About the only area where the supposedly more advanced version did better was not answering “sensitive” questions, or questions that might land Open AI in hot water, such as how to use AI to commit crimes.
“GPT-4 answered fewer sensitive questions from March (21.0%) to June (5.0%), while GPT-3.5 answered more (from 2.0% to 8.0%). It was likely that a stronger safety layer was likely to be deployed in the June update for GPT-4, while GPT-3.5 became less conservative,” according to the Stanford report.
The paper’s authors conclude that “users or companies who rely on LLM services as a component in their ongoing workflow… should implement similar monitoring analysis as we do here for their applications. To encourage further research on LLM drifts.”
Gary Marcus, a neuroscientist, author, and AI entrepreneur, told Defense One that the better lesson for the military is: stay away. “The real takeaway is that large language models are unstable; you can’t know from one month to the next what you will get out of them, and that means you can’t really hope to build reliable engineering on top of them. In sectors like defense, that’s a HUGE problem.”
Shortly after the paper came out OpenAI published a blog post describing how they were evaluating model changes between iterations. "We understand that model upgrades and behavior changes can be disruptive to your applications. We are working on ways to give developers more stability and visibility into how we release and deprecate models," it says.