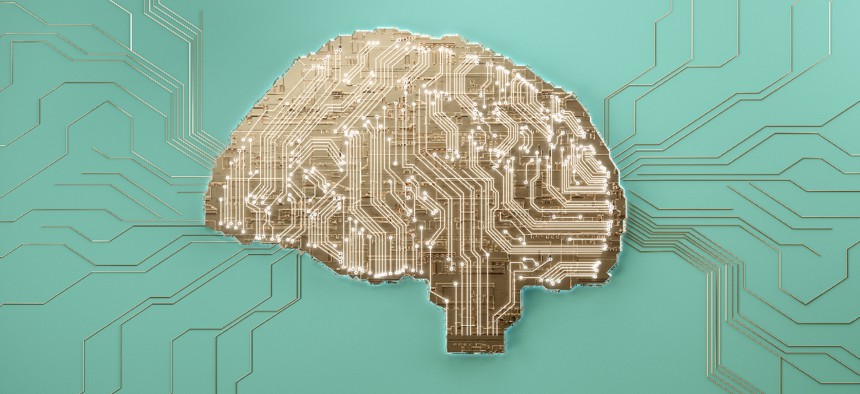
Andriy Onufriyenko/Getty Images
'Major Technical Challenges' Hinder Path to Responsible AI, NIST Official Says
Effective AI governance starts with developing metrics for trust—and that itself is fiendishly difficult.
New artificial intelligence tools that can perform complex tasks and chat with humans have exposed the lack of federal guardrails around the advancing technologies.
Short of comprehensive federal legislation, public and private entities have looked toward agencies like the National Institute of Standards and Technology—which recently released a new AI Risk Management Framework—for guidance on how to safely and effectively design and operate artificial intelligence.
Effective AI governance starts with developing metrics for trust, said Elham Tabassi, the chief of staff at NIST’s Information Technology Laboratory.
“If you really want to improve the trustworthiness of AI systems, any approach for risk management, any approach for understanding the trustworthiness, should also provide metrology for how to measure trustworthiness,” Tabassi said on Monday, speaking on a panel about AI governance.
Tabassi explained that AI systems are all about context, and how they work will change given the data they are analyzing. Assessments that analyze the risk in AI software should be tailored toward specific use cases and employ the proper metrics and test data to assess functionality.
“When it comes [to] measuring technology, from the viewpoint of ‘Is it working for everybody?’ ‘Is AI systems benefiting all people in [an] equitable, responsible, fair way?’, there [are] major technical challenges,” she said.
In both the AI RMF and separate Playbook released by NIST, these questions constitute the recommended socio-technical approach to building responsible AI systems. Developers and software designers helping an AI system’s creation should keep this approach in mind to prevent AI from being used in ways other than intended, according to the framework.
Tabassi added that marrying this approach with appropriate evaluation and measurement methods are “extremely important” to risk management when beginning to deploy a new system.
“It's important when this type of testing is being done, that the impact of community are identified so that the magnitude of the impact can also be measured,” she said. “It cannot be over emphasized, the importance of doing the right verification and validation before putting these types of products out. When they are out, they are out with all of their risks there.”
This advice comes as many private sector entities have rolled out AI-enabled capabilities for public experimentation, such as the recent deployment of Microsoft’s AI chat service combined with the Bing search engine. The software is only available to a limited number of users as software engineers work to scale and improve the current version.
Reports of strange and inappropriate communication from generative AI systems, namely from the Bing service enabled by OpenAI’s ChatGPT, have prompted lawmakers to introduce new regulatory legislation. President Joe Biden responded to the growing calls for better oversight into the development and usage of AI software with its AI Bill of Rights in October, but, like the NIST frameworks, the document is not a legal mandate.
Tabassi said she doesn’t think that this lack of formal regulatory laws in the U.S. will prevent improved AI use and development. Rather, she prioritizes the government spearheading and collaborating on international standards.
“My personal belief is that, regardless of the regulatory and policy landscape, having good, solid, scientifically-valid, technically-solid, international standards that talk about risks, that talk about risk management, that talk about trustworthiness, can be a good backbone and a common ground for all of these regulations and policy discussions,” she said.
NEXT STORY: The Reaper UAV Is Getting Its Own Drone Swarm